Machine Learning
حول هذه الدورة
تعلم الآلة (Machine Learning) هو أحد أهم وأشهر مجالات الذكاء الاصطناعي. تهدف هذه الدورة إلى تقديم فهم شامل لهذا المجال، بداية من تعريف تعلم الآلة وأهميته، وصولاً إلى تطبيقاته المتنوعة في حل المشكلات. خلال الدورة، ستتعلم كيفية بناء نماذج قادرة على محاكاة الذكاء البشري والتنبؤ بالنتائج بناءً على البيانات المدخلة. سيتم أيضاً توضيح الفرق بين التعلم الموجه (Supervised Learning) و التعلم غير الموجه (Unsupervised Learning)، بالإضافة إلى كيفية تطبيق كل منهما في حالات مختلفة.
أهداف التعلم
المواد
- Introduction to Machine Learning
- Data Preprocessing and Exploratory Data Analysis
- Supervised learning
- Regression
- Linear Regression, Polynomial regression
- Decision tree ,Random forest
- Classification
- Logistic Regression ,KNN, Naïve bay’s , SVM
- Decision tree, Random forest, XGBoost
- Dimensionality Reduction (Feature selection , Feature extraction)
- Unsupervised learning
- Clustering
- k-means , agglomerative, divisive , BDSCAN
- Recommendation systems, Apriori
- Model Evaluation, Training, validation, and testing sets
- Performance metrics (e.g., accuracy, precision, recall)
- Cross-validation, Hyperparameter tuning.
متطلبات
- Python: إتقان Python واستخدامه في بناء Machine Learning models.
- معالجة البيانات: القدرة على معالجة البيانات باستخدام مكتبات مثل Pandas و NumPy.
- الرياضيات الأساسية: إلمام بالمفاهيم الرياضية الأساسية اللازمة لتعلم Machine Learning.
الجمهور المستهدف
- طلاب الهندسة أو علوم الحاسب الذين يرغبون في التخصص في Machine Learning.
- المهنيون الذين يعملون في Data Science أو AI ويريدون تحسين مهاراتهم.
- المهتمون بتعلم كيفية تطبيق Machine Learning على مشاكل حقيقية واكتساب المهارات المطلوبة في هذا المجال.
منهاج دراسي
Machine Learning
1.Intro to Machine Learning00:13:33
2. Supervised Learning (Linear Regression)00:11:18
3. Supervised Learning (Gradient Descent )00:11:08
Quiz 1
4.Linear Regression Practice Part 100:19:35
5 .Linear Regression Practice Part 200:17:36
6.Linear Regression Practice Part 200:08:01
7. Linear Regression Practice (Part 3)00:10:35
8.Hypothesis Test00:09:20
9.Regression Evaluation Metrics00:08:52
Quiz 2
10.Polynomial Linear Regression00:10:18
11 Polynomial Regression (Part1)00:13:52
12. Polynomial Regression (Practice Part 2 )00:12:08
13.Polynomial Regression (Practice Part 3 )00:08:39
14.Polynomial Regression (Practice Part 4 )00:13:53
Quiz 3
15.Error in Machine Learning (Part 1)00:12:24
16 .Error in Machine Learning (Part 2)00:15:34
17.Underfitting and Overfitting (Part 1)00:15:04
18.Underfitting and Overfitting (Part 2)00:09:53
Quiz 4
19.Decision Tree (Part 1)00:19:23
20. Decision Tree (Part 2)00:11:48
21. Decision Tree (Part 3)00:07:25
22.Ensemble Models (Random Forest Regression )00:19:00
23.Ensemble Models (Gradient Boosting Regression )00:14:12
24.Decision Tree Practice (Part 1 )00:20:23
25.Decision Tree Practice (Part 2 )00:13:14
26.Decision Tree Practice (Part 3 )00:11:10
27.Decision Tree Practice (Part 4)00:07:56
28.Decision Tree Practice (Part 5)00:08:50
29.RandomForest Practice (Part 1)00:13:17
30.Random Forest Practice (Part 2)00:17:20
31.Ensemble Algorithms Practice (Part 1)00:17:57
32 Ensemble Algorithms Practice (Part 2)00:11:38
33.Ensemble Algorithms Practice (Part 3)00:10:31
34 Ensemble Algorithms Practice (Part 4)00:15:12
35.Ensemble Algorithms Practice (Part 5)00:05:58
36.Ensemble Algorithms Practice (Part 6)00:17:41
37.Ensemble Algorithms Practice (Part 7)00:11:11
38.Ensemble Algorithms Practice (Part 8)00:20:19
39.Ensemble Algorithms Practice (Part 9)00:14:20
40.Ensemble Algorithms Practice (Part 10)00:13:14
41. Ensemble Algorithms Practice (Part 11)00:09:12
42.Ensemble Algorithms Practice (Part 12)00:11:24
43.Ensemble Algorithms Practice (Part 13)00:18:51
Quiz5
44.Feature Selection Part 100:10:47
45.Feature Selection Filter Methods00:08:46
46.Feature Selection Wrapper Methods00:07:02
47.feature_selection00:13:56
Quiz6
48.Classification (logistic Regression)00:08:31
49.Logistic Regression (Part 2)00:04:37
50. Logistic Regression (Part 3)00:09:35
51.Logistic Regression (Part 4)00:09:04
52.Logitic Regression Practice (Part 1)00:05:25
53.Logitic Regression Practice (Part 2)00:12:14
54.Logitic Regression Practice (Part 3)00:10:45
55.Logitic Regression Practice (Part 4)00:08:51
56.Logitic Regression Practice (Part 5)00:07:43
57.Logitic Regression Practice (Part 6)00:10:13
58.Logitic Regression Practice (Part 7)00:12:15
59.Logitic Regression Practice (Part 8)00:09:07
60.Logitic Regression Practice (Part 9)00:05:14
61.Logitic Regression Practice (Part 10)00:07:16
62.Logitic Regression Practice (Part 11)00:11:03
63.Logitic Regression Practice (Part 12)00:14:13
64.Logitic Regression Practice (Part 13)00:00:21
Quiz 7
65.Classification Evaluation Metrcis00:21:44
Quiz 8
66.Decision Tree (Part 1)00:13:46
67.Decision Tree (Part 2)00:13:46
68.Decision Tree (Part 3)00:06:01
69.Random Forest00:07:49
70.Decision Tree Practice (Part 1)00:31:27
71.Decision Tree Practice (Part 2)00:16:29
72.Decision Tree Practice (Part 3)00:10:49
73.Random Forest Practice00:18:48
Quiz 9
74.Deal with Imbalance Data (Part 1)00:21:06
75.SMOTE00:16:03
76. Naive Bay’s00:18:03
77.Practice (SMOTE-Naive Bay’s)00:20:06
78.Unsupervised Learning00:26:40
79.K-Means00:16:15
80.Hierarchical Clustering00:17:20
81.Density Based Clustering00:11:36
82.Clustering Practice (Part1)00:13:23
83.Clustering Practice (Part2)00:11:45
Quiz 10
84.Association Rule00:11:35
85.Apriori00:09:28
86.Apriori Practice (Part 1)00:23:18
87.Apriori Practice (Part 2)00:4:26
Quiz 11
احصل على شهادة
أضف هذه الشهادة إلى سيرتك الذاتية لإثبات مهاراتك وزيادة فرصك في لفت الانتباه.
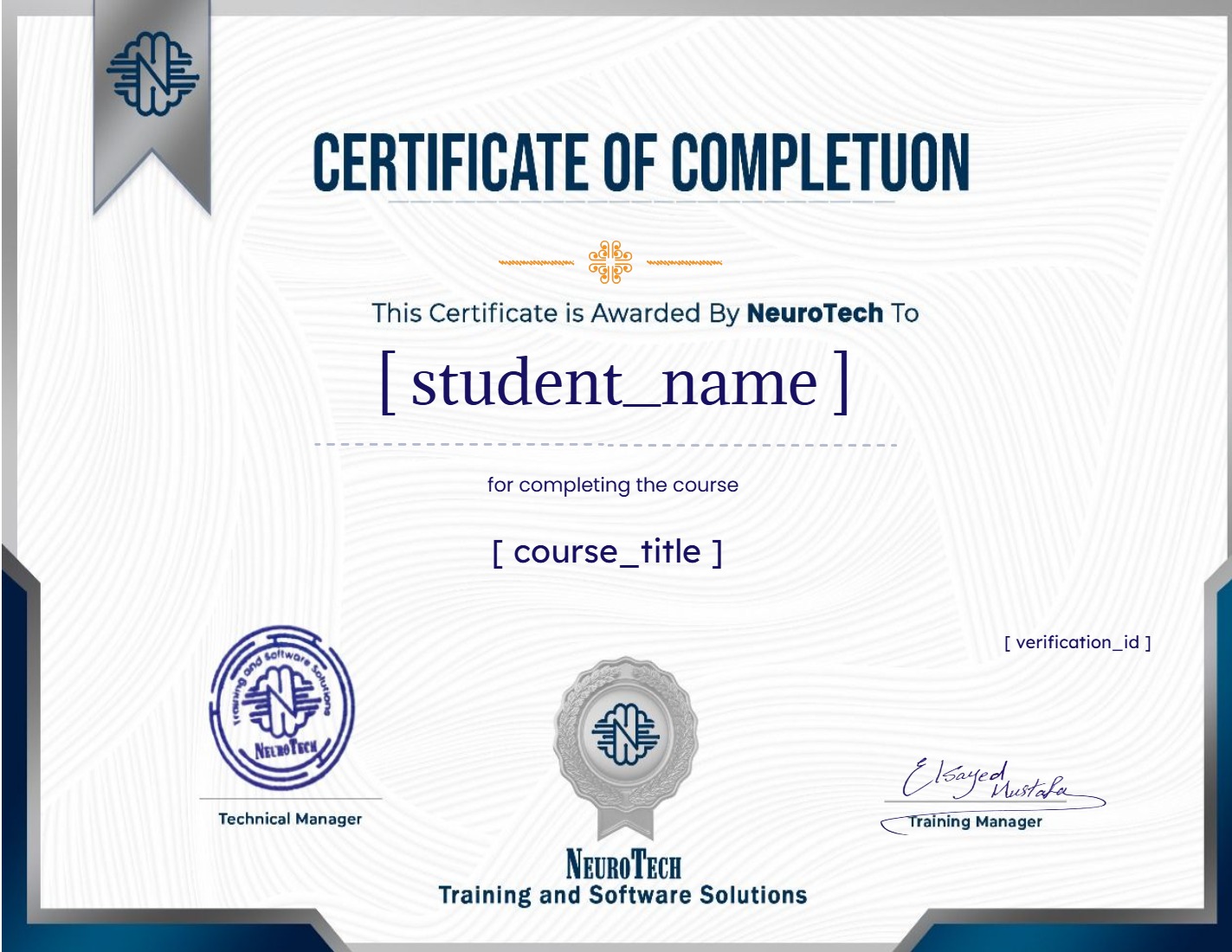
أساتذتك
Ali Elshenawy
Artificial intelligence developer
My name is Ali Elshenawy, and I am a passionate computer science graduate with a Bachelor's degree from Mansoura University. I graduated with excellent with honor degree in 2020, and my graduation project was focused on Drowsiness detection using deep learning and computer vision. After that I finished my pre-master in 2021 from Mansoura university and my graduation project was focused on Breast cancer diagnosis using machine learning. After that, I pursued a Master's degree in Engineering in the field of Data Science and AI from the University of Ottawa in Canada, from 2022 to 2023. My graduation project was "Automatic mixed Arabic-English speech summarization", where I used sequence to sequence models to create a unique and effective solution.
In addition to my academic qualifications, I have earned several certificates in the field of AI and data analytics, including HCIA,HCIP in AI from Huawei, IBM Data analytics, and IBM Data Engineer. I have also been a part of a team that developed a chatbot that answers questions.
now I work as a senior Data scientist at I-Score and Team leader instructor in Artificial intelligence at Amit learning. and teach that fields (python, data science, ml, dl, NLP, CV, MLOPS)
My passion for the field of AI and data science, combined with my academic qualifications and hands-on experience, make me an ideal candidate for any organization looking for a talented and motivated individual to join their team.