Machine Learning
حول هذه الدورة
Machine Learning is one of the most important and well-known fields of Artificial Intelligence. This course aims to provide a comprehensive understanding of this field, starting from defining Machine Learning and its significance to exploring its various applications in problem-solving. Throughout the course, you will learn how to build models capable of simulating human intelligence and predicting outcomes based on input data. The course will also explain the difference between Supervised Learning and Unsupervised Learning, as well as how to apply each in different scenarios.
أهداف التعلم
Requirements
- المعرفة الأساسية: من الأفضل أن تكون لديك معرفة أساسية بالرياضيات (خصوصًا الجبر والإحصاء) وبرمجة Python.
- أدوات مطلوبة: جهاز حاسوب مع اتصال بالإنترنت وبرنامج Python مُثبت عليه مكتبات مثل Scikit-learn وTensorFlow.
- المهارات البرمجية: الدورة موجهة للمبتدئين في التعلم الآلي، لكن معرفة أساسية بـ Python هتكون مفيدة.
- تطبيق عملي: الدورة هتتضمن تمارين عملية لبناء نماذج تعلم آلي وتطبيقها على مجموعات بيانات حقيقية.
الجمهور المستهدف
- الطلاب: طلاب الهندسة أو علوم الحاسب أو الرياضيات الذين يرغبون في التخصص في الذكاء الاصطناعي وتعلم الآلة.
- Professionals: Engineers or analysts who want to gain deeper knowledge in how mathematics can be used to improve the performance of computer models.
- محللو البيانات: الذين يريدون إضافة مهارات التعلم الآلي لتحليل البيانات وإيجاد حلول ذكية.
- المطورون: الذين يرغبون في تعلم كيفية بناء وتطبيق نماذج تعلم آلي باستخدام Python.
- الطلاب: الذين يدرسون علوم البيانات أو الذكاء الاصطناعي ويرغبون في تعلم الأساسيات.
- المهتمون بالتقنيات الحديثة: أي شخص مهتم بمجال التعلم الآلي ويريد تطبيقه في حياتهم المهنية أو الدراسية.
منهاج دراسي
Machine Learning
Introduction to Machine Learning03:05:15
Quiz1
Linear Regression01:30:26
Quiz2
Polynomial Regression03:28:24
Quiz3
Linear Regression (Practice)02:40:48
Quiz4
Ploynomial Regression (Practice)02:42:10
Quiz5
Underitting Vs Overfitting02:51:28
Quiz6
Decision Tree02:50:03
Quiz7
Decision Tree (Practice)03:10:07
Quiz8
Ensemble Models Practice (Gradient Boosting & Random Forest)03:34:59
Quiz9
Feature Selection03:09:50
Quiz10
Logistic Regression02:17:51
Quiz11
Decision Tree Classification02:52:50
Quiz12
Dealing with Imbalance Data02:43:50
Quiz13
XGBoost Classifier03:19:44
Quiz14
Support Vector Machine03:03:30
Quiz15
Naive Bayes02:46:36
Quiz16
Unsupervised Learning02:51:07
Quiz17
Association Rules03:27:27
Quiz18
احصل على شهادة
أضف هذه الشهادة إلى سيرتك الذاتية لإثبات مهاراتك وزيادة فرصك في لفت الانتباه.
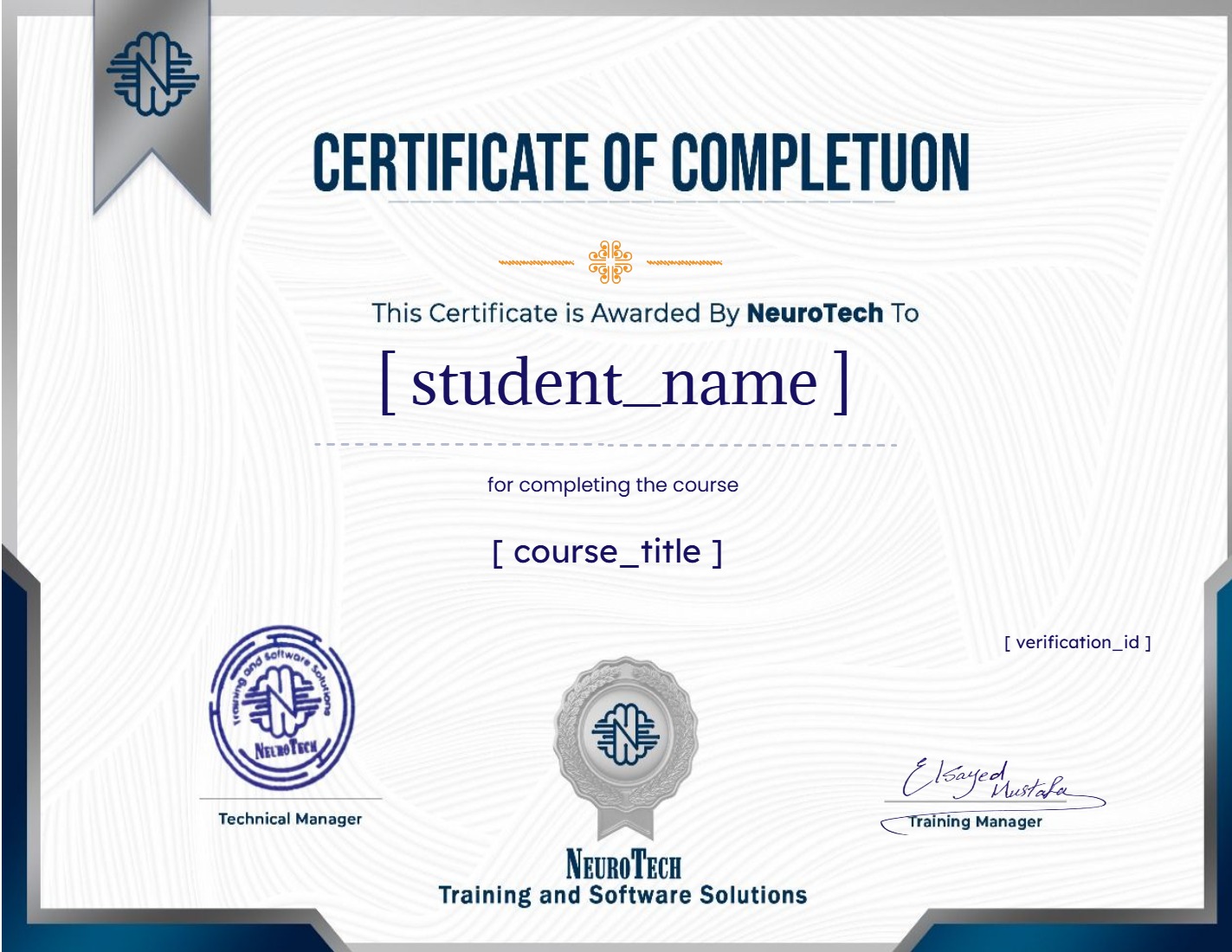
أساتذتك
Ahmed Ashraf
Machine Learning Engineer @ Cybral
Results-driven Machine Learning Engineer with expertise in NLP and MLOps, specializing in optimizing model performance, developing scalable data solutions, and enhancing system security. Skills: • Machine Learning & NLP: supervised, unsupervised algorithms, NN, CNNs, RNNs, LSTMs, Classical NLP, Tokenization, stemming, lemmatization, POS tagging, named entity recognition (NER), Embeddings, Word2Vec, GloVe, BERT, GPT, Attention, Transformer, Pytorch, HuggingFace, LLMs, Prompt Engineering, RAG, Vector Store, Langchain, LLM serving • MLOps & Deployment: Azure Repos - Pipelines, Git, Docker - Compose - Swarm, Mlflow, Weights & Biases, OLLAMA • API Development & Integration: Python, Flask, Flask-RESTx, Http/s, GRPC, Protocol Buffers, Swagger, Postman, SSL/TLS, Authentication, role-based access control, rate limiting, Microservices, Service Registry • Graph Database: Neo4j, Cypher language, APOC: Awesome Procedures on Cypher • Other Tools & Technologies: Numpy, Pandas, Matplotlib, Sklearn, NLTK, Redis, A/B Testing